Financial Markets in the Age of AI: Opportunities and Challenges
Financial Markets in the Age of AI: Opportunities and Challenges
The AI boom is transforming all industries. The financial industry is no exception. AI is increasingly prevalent in financial services and is reshaping traditional institutions by creating more efficient operating models. This article explores the opportunities AI could offer financial markets and the challenges it presents to different stakeholders.
Opportunities
In trading, AI tools can help companies execute processes quickly and efficiently. Traders must consider various factors, such as the economy, market, and strategies, to make informed decisions and accurate predictions. The implementation of AI can assist in this process by compensating for the limitations of traditional investment models.
Big Data and Machine Learning
These AI algorithms use machine learning technology to analyze vast amounts of data, such as stock prices, news articles, macroeconomic indicators, and sentiment analysis. Unlike human analysis, which can be limited by incomplete data, latency, or inaccurate results, AI algorithms can process millions of data points in just a few seconds. They can mobilize various data sources, analyze different types of data simultaneously (such as market prices, news, social media posts, satellite images, etc.), and identify patterns and complex correlations between multiple variables. These correlations can be difficult for human traders to assess.
By utilizing statistical methods, algorithms can analyze large volumes of historical and real-time data to identify upward and downward trends. This allows for more accurate predictions of future market movements. Additionally, these AI algorithms can automatically derive rules and continuously learn to fine-tune their capabilities for an enhanced, data-driven decision-making process.
High-frequency trading and Quants
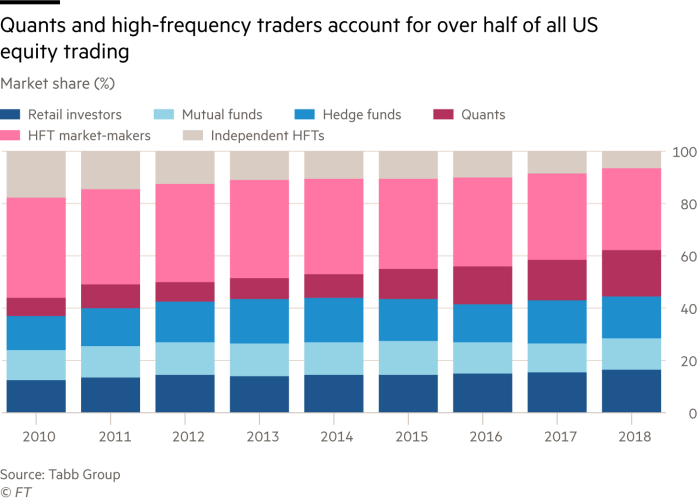
The impact of Al algorithms is eminent in high-frequency trading (HFT). In fact, there has been a race between HFT companies for increased automation that would allow them to minimize their execution and response time to nanoseconds. This is why it heavily relies on the use of AI.
When deployed at all stages of trade, AI can allow HFT participants to benefit from an autonomous value chain, thus extremely reducing execution timeframes. For instance, these sophisticated technologies can swiftly detect signals, design strategies, and execute trades to capture undiscovered opportunities and capitalize on minor inconsistencies across markets. This can be achieved by trading at a rapid pace that can only be maintained by machines.
Operational Efficiency
The use of automation and computerized business operations has enabled many companies across various industries to reduce operational costs and increase productivity, leading to improved operational efficiency and profitability. This trend is also evident in financial institutions.
Goldman Sachs’s U.S. cash equities trading desk in New York has seen a significant reduction in employed traders over the past two decades. In 2000, the desk had 600 traders, but by 2017, that number had decreased to just two. This reduction is due to the increasing adoption of automated trading programs. Goldman Sachs has been intensifying its use of AI-driven programs, expanding from equities to forex and, more recently, derivatives markets. The investment bank is using neural networks, an AI method that imitates the human brain, to develop its software.
Processes are becoming increasingly automated, from front-office to back-office operations. Nasdaq’s new exchange AI-powered order type has improved fill rates by 20.3% and reduced markouts by 11.4%. AI can also help minimize losses related to operational risks, such as human error and fraud. Blackrock transformed its risk management function, a cost center by default, into a revenue stream by launching Aladdin, an AI-based software for risk assessment and portfolio management.
Challenges
Generative AI has become ubiquitous, from simple text generation to automated code testing. The market is projected to reach US$44.89 billion in 2023, with a CAGR of 24.4%. However, major banks and financial services firms such as JP Morgan Chase, Citibank, and Bank of America are currently banning the use of ChatGPT.
Traditional and generative AI can pose a risk to financial institutions. This is because many innovative solutions are provided by external vendors. If these companies do not conduct the necessary due diligence in advance, implementing these solutions in their day-to-day operations can have profound implications for data privacy. The technology uses inputs from all users to fine-tune its capabilities, detect patterns, and draw inferences. Therefore, these risks are inherent to the technology. Data leakage, such as proprietary information, can have a severe impact on both organizations and investors. Therefore, it is important to minimize the probability of such incidents occurring.
Last May, a falsified image of an explosion near the Pentagon moved markets. The AI-generated image had set off fears, which brought US stocks down. This event has shown how this technology can be a threat, as some actors may try to take advantage of the vulnerability of financial markets. The fraud scheme could extend to other kinds of spoofing, such as voice cloning, to further manipulate the markets. As new tools emerge, scrutiny should be heightened since cybersecurity is at stake and misinformation can easily feed investors panic, thus disrupting the market.
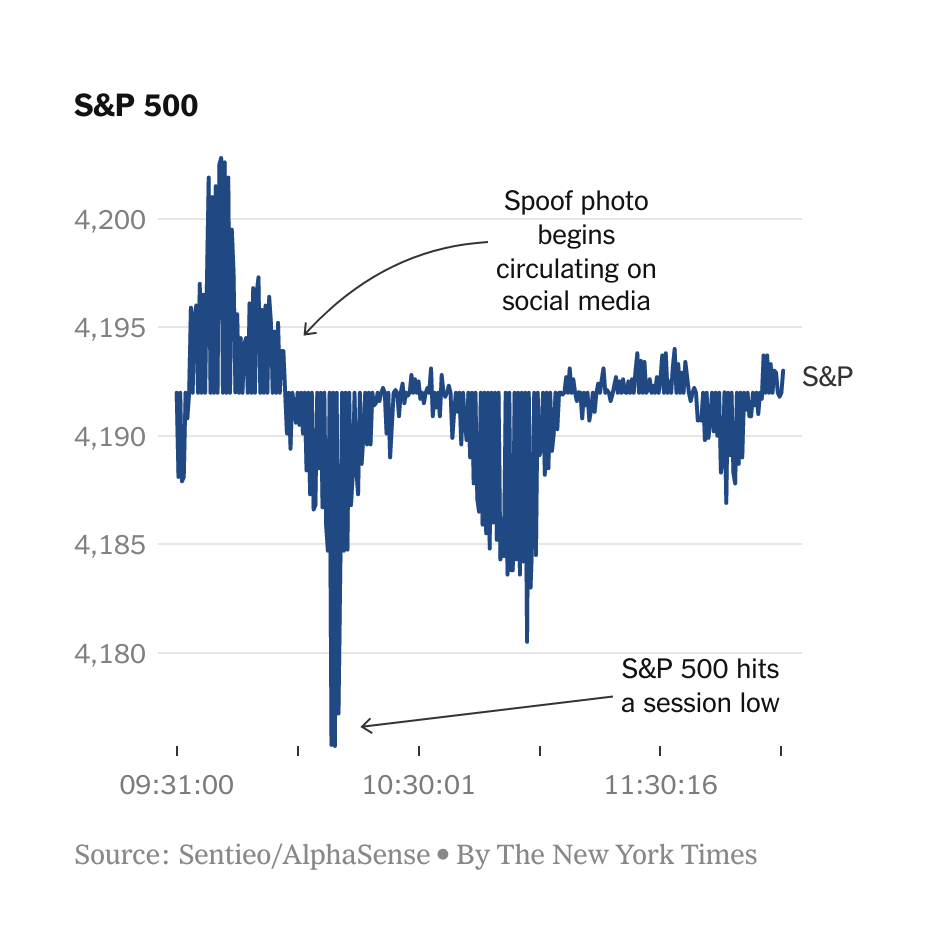
Furthermore, the financial landscape is highly interconnected, and AI systems are interdependent. Therefore, as the financial industry adopts this technology, it could significantly expose itself to systemic risks by exacerbating biases, like herding behaviors, or by producing inaccurate results, also known as AI hallucinations.
Financial institutions should be cautious about implementing third-party AI-based models due to transparency concerns. The architecture of algorithms can be very complex and can include an extensive number of parameters. It is a requirement for financial institutions to be able to explain the rationale behind their decisions to all stakeholders. Therefore, it is a new challenge for these organizations to grasp how these models work and generate their outputs.
Conclusion
AI is transforming financial institutions and the products and services they offer to clients. This includes chatbots, automated trading algorithms, and other innovations that enhance the customer experience. However, AI can also be disruptive to markets, posing financial and operational risks to all stakeholders. Regulators should focus on creating a framework that ensures the security, transparency, robustness, and stability of the financial landscape. Control functions must deepen their understanding of AI and its regulatory implications to design effective risk management practices.
Sources
- https://mpra.ub.uni-muenchen.de/118175/1/Use%20of%20AI%20in%20Stock%20Trading.pdf
- https://www.ft.com/content/fdc1c064-1142-11e9-a581-4ff78404524e
- https://www.oecd.org/finance/financial-markets/Artificial-intelligence-machine-learning-big-data-in-finance.pdf
- https://www.technologyreview.com/2017/02/07/154141/as-goldman-embraces-automation-even-the-masters-of-the-universe-are-threatened/
- https://www.statista.com/outlook/tmo/artificial-intelligence/generative-ai/worldwide
- https://finance.yahoo.com/news/companies-now-banning-workers-using-164126139.html
- https://www.nytimes.com/2023/05/23/business/ai-picture-stock-market.html
- https://www.nasdaq.com/press-release/nasdaq-announces-first-exchange-ai-powered-order-type-approved-by-the-sec-2023-09-08
- https://www.imf.org/-/media/Files/Publications/FTN063/2023/English/FTNEA2023006.ashx#:~:text=Cybersecurity%20of%20GenAI%2C%20including%20potential,for%20example%2C%20in%20bank%20runs.