How to Prioritize Your AI Analytics and Automation Roadmaps
How to Prioritize Your AI Analytics and Automation Roadmaps
Artificial intelligence (AI) enables companies to automate their workflows, predict future outcomes, and enhance productivity. Organizations can incorporate this technology for their analytics and other essential tasks by creating a detailed and systematic automation roadmap.
Although automation roadmaps can be implemented across a company, they are usually resource-intensive. Therefore, businesses must redesign their automation strategies to prioritize the most effective use cases for AI technologies based on their targets and resources.
This article covers the role of AI analytics and strategic roadmaps in organizations. It also highlights valuable frameworks for prioritizing an AI roadmap, the steps for building an effective AI adoption strategy, AI analytics use cases, and implementation best practices for automated roadmaps. It also discusses how organizations can position themselves for future advancements in AI analytics.
Enhance Client Outputs with AI
Discover how our data analytics team leverages advanced AI models across sectors
Download eBook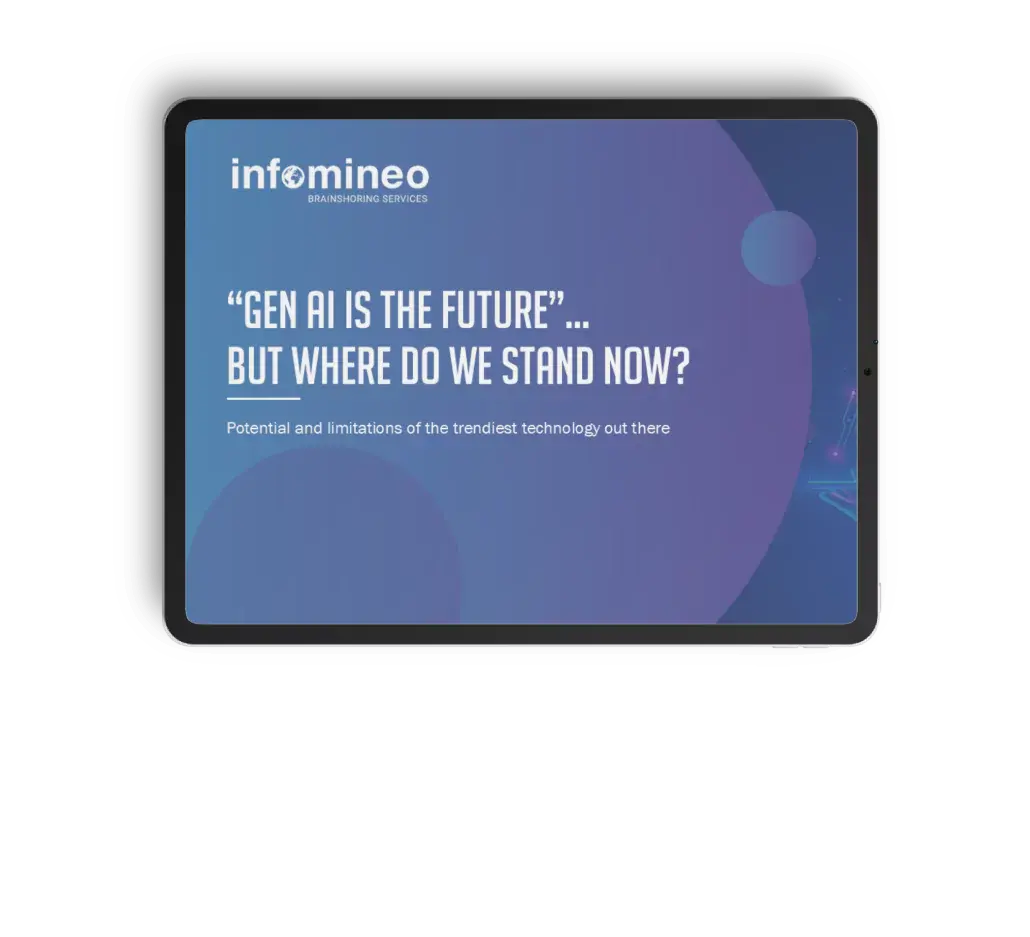
Introduction
AI analytics harnesses artificial intelligence for data-driven decision-making. It involves using advanced algorithms to automate several aspects of a company’s data analytics exercise. For example, organizations can use this technology to interpret their datasets, discover hidden trends, and generate recommendations.
Understanding AI analytics
Modern businesses can leverage AI analytics to augment their existing data processes and improve the efficiency and accuracy of their data management practices. Organizations can also incorporate AI algorithms to build predictive models depending on the nature of their projects.
AI analytics includes several components, including data collection, data cleaning, natural language processing (NLP), advanced data visualization, natural language generation (NLG), statistical analysis, and predictive model optimization. Furthermore, many AI frameworks can be integrated with established workflows and software.
The Need for Strategic Roadmaps
AI analytics is a fast-growing technology with expanding use cases in various industries. 65% of respondents in the 2024 McKinsey Global survey reported the use of AI in their organizations. This survey also revealed that most companies spend more than 20% more on analytical AI than generative AI, highlighting the need for automation in data analytics processes.
Organizations must evaluate their available use cases of AI analytics technologies and determine the most effective choice to optimize their output. Automation roadmaps provide a blueprint for the implementation of AI analytics. They enable companies to allocate resources efficiently based on short- and long-term goals to achieve maximum ROI.
Automation roadmaps also allow organizations to carefully integrate AI tools into their operational workflow with minimal downtimes. A well-structured strategy is critical for businesses to guarantee a seamless transition to newer technologies without disrupting their operations.
Assessing Current State
Businesses seeking to effectively allocate their resources and prioritize their AI analytics and automation roadmaps must begin by thoroughly assessing their operations. This involves two critical steps.
Evaluating Existing Analytics Capabilities
A company’s existing analytics framework significantly determines the extent of its automation exercise. Implementing an automation roadmap demands an inventory of available tools and technologies. Companies must also evaluate their data quality and availability and identify gaps in their analytics processes.
Identifying Business Objectives
Businesses must identify their objectives to ensure their automation roadmap achieves the highest ROI. Organizations can create long- and short-term goals that drive their AI analytics implementation using key performance indicators. Furthermore, stakeholder analysis and engagement are critical in establishing corporate targets and formulating a practical automation roadmap.
Prioritization Framework
Prioritization frameworks guide the implementation of automation and AI analytics. There are two factors to consider when developing a framework for incorporating AI technologies to enhance decision-making and achieve corporate targets.
Value vs. Effort Matrix
A value vs. matrix is a decision-making framework used to evaluate and rank corporate tasks based on their value and the resources required for each activity. It is a 2 x 2 matrix that organizes activities into one of four categories:
1. High-value high-effort
2. High-value low-effort
3. Low-value high-effort
4. Low-value low-effort
Companies can adopt this matrix to assess the impact of several AI analytics initiatives and determine the most profitable application of this technology. High-level management and project management experts can benefit from this framework as it is easy to interpret and offers clear visualization.
Value vs. Effort matrices also allow organizations to estimate the complexity of their AI analytics goals and create an effective roadmap for implementing AI in their operational workflow. By adopting a value vs. effort prioritization framework, businesses can identify tasks that will deliver optimal returns while de-emphasizing low-value, high-effort activities.
Quick Wins vs. Long-Term Investments
Another effective strategy for businesses prioritizing their AI analytics and automation roadmaps is organizing their projects into quick wins and long-term investments. Quick wins are tasks that can be completed relatively quickly with a positive impact on essential KPIs.
Long-term investments, however, are critical to a business’s stability and scalability and a core aspect of corporate strategy. Categorizing activities into both categories enables companies to balance the need for short-term gains with their longer-term strategic goals.
An effective automation roadmap facilitates growth momentum by identifying low-hanging fruits for AI implementation while ensuring adequate resource allocation toward other significant future projects.
AI Analytics Use Cases
AI analytics is a valuable tool with several use cases in the retail, finance, healthcare, energy, marketing, and manufacturing industries. Organizations can harness the potential of advanced, self-learning algorithms via predictive analysis, natural language processing, and computer vision.
Predictive Analytics
Predictive analytics uses mathematical models to forecast future events. AI analytics can be implemented to analyze historical datasets to discover patterns, predict trends, and solve business problems.
Depending on the nature of an analytics project, AI analytics tools can use classification, clustering, or time series models to enhance data-driven decision-making. Companies can apply an automation roadmap to gradually incorporate predictive analytics models across various departments and achieve their strategic KPIs.
AI analytics can be used for predictive maintenance, supply chain optimization, and user behavior prediction. It is also a vital tool for highlighting high-impact initiatives. For example, healthcare companies can harness machine-learning models to predict patient populations with a low survival rate and formulate intervention strategies to decrease mortality statistics.
Natural Language Processing
Natural language processing (NLP) is a subset of AI that enables software to understand, review, and communicate using human language. NLP has a market size of $29 billion and is one of the most popular use cases for AI analytics.
Companies implement NLP algorithms to gather and analyze large volumes of text and speech data. AI analytics with NLP can be applied to build virtual assistants and chatbots. It can also be used to conduct sentiment analysis and generate insights from unstructured datasets.
For example, using NLP, marketing companies can collect and analyze information from social media to conduct product market research and identify user pain points. This technology saves organizations time and resources by eliminating manual text analytics tasks and ensuring more accurate datasets.
Computer Vision
Computer vision is another domain in AI analytics that uses images and videos to train models. Computer vision algorithms can identify visual data using prebuilt image tagging, optical character recognition (OCR), and responsible facial recognition. They can also classify these image and video data and generate predictions.
This application of AI has been implemented in many industries to enhance user experiences, uncover patterns, and automate decision-making. For example, manufacturing companies can use this computer vision to sort their products.
AI analytics tools can be incorporated into quality assurance, enabling businesses to flag defective items in a production line and ensure corrections. Government agencies can also use computer vision for security and surveillance and to assess infrastructure to determine upcoming maintenance projects.
Automation Opportunities
Organizations can adopt automation roadmaps using several approaches. Two of the common opportunities for automation include process and decision automation.
Process Automation
Process automation involves implementing digital solutions for repetitive tasks. Companies can build their roadmaps by identifying routine activities and creating algorithms to complete these responsibilities.
Robotic process automation (RPA) can perform system navigation, data discovery and extraction, and analysis of various file formats. Implementing process automation allows organizations to streamline their operations and maximize their output. It also reduces human errors and facilitates productivity by enabling employees to focus on core responsibilities.
Several organizations use RPA to improve their recruitment, employee onboarding, and customer service practices. Businesses can also engage RPA to speed up their data analytics workflows by leveraging AI and machine learning technologies to obtain and analyze big data.
Decision Automation
Decision automation is a process that relies on AI, big data, and business intelligence to automate decision-making. Decision automation allows AI-powered decision-making systems to harness available datasets and determine an appropriate action.
Companies depend on decision automation to improve their productivity, guarantee consistency in decision-making, and eliminate human errors. It is often applied to routine operations and influenced by preset business guidelines, available data, or both.
AI-based decision systems offer varying levels of automation, depending on whether an organization opts for a fully automated or hybrid approach. For example, decision automation can provide support via automated reports and insights based on real-time information. However, decision automation may incorporate predictive modeling to forecast future outcomes and respond effectively.
Building the Roadmap
Building an automation roadmap demands careful consideration of several factors, including adaptability and availability of resources. Organizations must create a roadmap that supports seamless integration without disrupting the existing operations workflow or compromising their corporate targets.
Phased Approach
AI roadmaps must adopt a strategic, phasic approach considering long, mid-, and short-term business objectives. A phased AI roadmap has a preset timeline with clearly defined milestones to track the progress of AI implementation. It guarantees measurable short-term results while optimizing organizational workflows for faster achievement of future corporate goals.
Companies building an AI implementation blueprint can quickly incorporate this technology for basic daily operations to boost staff productivity. Short-term progress with AI automation can demonstrate its viability to stakeholders and employees and facilitate its adoption and use cases across departments.
However, organizations must also optimize their roadmaps for greater AI involvement in complex automation tasks such as predictive modeling and fully automated decision-making for core business problems. A phased approach enables companies to gradually expand their use of AI analytics and other AI-powered technologies while rebuilding their work culture and preserving their advantage in a competitive market.
Resource Allocation
Businesses must create an AI roadmap that optimizes their available financial resources and personnel. Therefore, building an automation blueprint requires identifying the necessary infrastructure, skill sets, and technology.
Depending on the company’s staff competency, they may need to budget for periodic training to bridge their employee’s skill gap with AI tools. Creating a practical AI roadmap will involve thoroughly evaluating an organization’s available resources and financial strength to develop a strategy that achieves the highest ROI.
Implementation Best Practices
Organizations can adopt agile implementation, change management, and governance principles to guarantee seamless integration of AI technologies and compliance with data handling procedures.
Agile Implementation
Integrating Agile principles for AI analytics and automation promotes faster implementation and ensures maximum ROI. Organizations must use a flexible and iterative approach to develop and deploy their AI technologies.
Agile strategies rely on collaboration and continuous feedback to guarantee a minimum viable product (MVP) as fast as possible. This enables businesses to accumulate quick wins while incrementally increasing their level of automation and the complexity of their models.
Furthermore, Agile implementation involves constantly monitoring KPIs to evaluate AI technologies’ impact on long- and short-term corporate goals.
Change Management
Effective change management strategies reduce resistance and increase AI adoption across various units. Successful AI adoption is measured by an organization’s acceptance and use of automation technologies. AI can be disruptive to a business’s established daily workflow. Therefore, employees may be reluctant to incorporate this solution into their tasks.
Change management enables companies to assess the potential impact of implementing an automation roadmap and create an integration plan. It also involves establishing systems to promote lasting employee adoption.
These strategies may include building feedback structures, encouraging open communication, and providing frequent training and upskilling programs to manage resistance at all levels.
Governance and Ethics
AI governance and ethics is a significant concern for governments and businesses. Organizational automation blueprints must establish an AI ethics guideline that ensures data privacy, security, accountability, and reliability.
Automation and AI analytics must adopt a human-centric approach that guarantees the protection of the end users. Therefore, companies seeking to integrate AI into their workflows must comply with the data privacy regulations from relevant authorities such as the General Data Protection Regulation (GDPR).
Measuring Success
Businesses can track the success of their automation process by evaluating two critical criteria – KPIs and ROI. These indices can be used to assess the effectiveness of an AI implementation strategy and determine areas of improvement.
KPI Tracking
KPIs are a reliable method for defining the success of an AI implementation strategy. Organizations can use predefined metrics to monitor the effectiveness of their AI analytics technologies and their impact on short- and long-term goals.
KPIs can be visualized and tracked in real time using dashboards. Stakeholders can use these dashboard reports to fine-tune their AI roadmaps for optimal performance.
ROI Analysis
Companies can calculate the ROI on automation projects based on key metrics such as costs and measurable and immeasurable benefits. Automation roadmaps often include training, software, infrastructure, and other additional expenditures. Furthermore, implementing these technologies usually yields benefits such as saved time and person-hours.
Measuring the success of an AI implementation blueprint involves considering these factors and performing a long-term impact assessment to determine the roadmap’s sustainability.
Future-proofing Your Roadmap
AI analytics is an evolving field. Organizations must adapt their automation roadmaps to accommodate new and emerging technologies and promote scalability.
Emerging Technologies
Companies looking to integrate advanced algorithms and AI for their analytics and other operational workflows must remain updated with the latest trends. Innovations such as multimodal AI, quantum computing, edge AI, and the increased popularity of open-source AI resources have the potential to shape the application of AI for analytics in the future.
Therefore, an AI roadmap must monitor the progress of these advancements and prepare to integrate them depending on the company’s requirements.
Scalability and Flexibility
AI implementation is highly dependent on architecture and infrastructure. Future-proof automation roadmaps ensure the creation of AI systems that are readily scalable and flexible. Implemented AI-based solutions must allow constant adaptation and improvement for application across various use cases.
Infomineo – Your Partner in AI Analytics and Automation Roadmaps
At Infomineo, we specialize in helping organizations harness the power of AI analytics and automation to streamline workflows, predict future outcomes, and enhance productivity.
Our team provides expert guidance and tailored strategies to prioritize and implement AI technologies effectively, ensuring your business achieves maximum ROI and remains competitive in an evolving market.
Leveraging our extensive experience, we assist you in developing comprehensive automation roadmaps, assessing current capabilities, defining business objectives, and integrating cutting-edge AI tools. Let us help you build a future-proof AI strategy that drives innovation and positions your organization for long-term success.
FAQ
What is an automation roadmap?
An automation roadmap is a strategic plan that outlines how a company will implement automation technologies, including AI, to enhance its operations. It details the steps, resources, and timelines needed to achieve specific automation goals.
Why are automation roadmaps resource-intensive?
Automation roadmaps are resource-intensive because they require significant investments in technology, training, and change management. Implementing AI solutions often involves complex integration with existing systems and processes, which can be costly and time-consuming.
How can businesses prioritize AI use cases?
Businesses can prioritize AI use cases by evaluating their potential impact and the resources required. Frameworks such as the value vs. effort matrix help organizations categorize projects based on their value and effort, allowing them to focus on high-value, low-effort initiatives first.
What are some common AI analytics use cases?
Common AI analytics use cases include predictive analytics, natural language processing (NLP), and computer vision. These technologies are used in various industries for tasks like forecasting trends, automating customer service, and improving quality control in manufacturing.
How can companies ensure successful AI implementation?
Successful AI implementation requires a phased approach, adequate resource allocation, and adherence to best practices like agile methodologies, change management, and governance. Continuous evaluation and adjustment of the AI roadmap are essential to ensure it meets the organization’s goals.
What are the benefits of using a value vs. effort matrix?
A value vs. effort matrix helps organizations prioritize AI projects by assessing their potential benefits against the effort required. This approach ensures that resources are allocated to initiatives that offer the highest return on investment, enabling more efficient and effective implementation of AI technologies.
How can AI analytics improve decision-making?
AI analytics enhances decision-making by providing data-driven insights, predicting future outcomes, and identifying trends. By leveraging advanced algorithms, businesses can make more informed decisions, reduce uncertainty, and optimize their operations.
What is the role of change management in AI adoption?
Change management is crucial in AI adoption as it helps address employee resistance and ensures smooth integration of new technologies. Effective change management strategies include clear communication, training programs, and establishing feedback mechanisms to support staff throughout the transition.
Why is governance important in AI analytics?
Governance ensures that AI analytics is implemented ethically and in compliance with regulations. It involves setting guidelines for data privacy, security, accountability, and reliability, ensuring that AI solutions are used responsibly and protect end-users’ interests.
How can companies future-proof their AI roadmap?
To future-proof their AI roadmap, companies should stay updated with emerging technologies, ensure scalability and flexibility in their AI solutions, and be prepared to integrate new advancements as they arise. This approach helps organizations maintain a competitive edge and adapt to technological changes.
Conclusion
Creating an AI implementation strategy is vital for efficient allocation of resources and promoting increased adoption of AI technology for operations. Companies building an AI roadmap must assess their current state by examining their existing analytics capabilities and outlining their business objectives.
AI roadmaps also require a prioritization framework and the knowledge of implementation best practices such as the incorporation of agile principles, application of change management strategies, and compliance with governance and ethical regulations.
Integrating AI into your organization’s workflow is a gradual process that requires continuous evaluation and adjustments. You must measure the impact of your AI adoption strategy by tracking KPIs and evaluating its long-term ROI.
AI analytics and other AI-based trends are experiencing improved adoption across various industries. Companies seeking to thrive and maintain their competitive advantage must create an AI roadmap that achieves a maximum ROI and supports their long- and short-term goals.