Power of Data: Navigating Big Data Analytics for Business Excellence
Power of Data: Navigating Big Data Analytics for Business Excellence
Big data is primarily defined by three V’s: Volume, Velocity, and Variety. It involves analyzing massive volumes of datasets[U91] , coming at ultra-fast speeds (Velocity), from a variety of sources, including social media, sensors, and electronic financial transactions. Big Data Analytics is the process of inspecting, modifying, eliminating errors, and interpreting vast and intricate data sets to reveal patterns, trends, and valuable insights that businesses can capitalize on to make informed decisions and grow.

Core Components of Big Data Analytics Services
There are key components and stages at the heart of big data analytics services that define the productive outcomes and benefits businesses enjoy. These include the following:
Data Sources and Storage
Collecting data is the primary step in data analytics services. Once data is gathered from sources such as social media, research publications, and sensors, it should be stored in a safe repository for easy access and then processed and analyzed for accurate decision-making.
Data Processing
Data processing is the refinement and restructuring of data to present it in an easily understandable manner. It involves the following steps:
- Organization: Arranging data into categories
- Cleaning: Eliminating inconsistencies and flaws
- Integration: Assembling data from varied sources
- Transformation: Presenting data in a consistent form
Machine Learning and Predictive Analytics
Machine learning relies on algorithms to observe, study, and memorize patterns in large data sets. Via automated data analytics, machine learning software can learn patterns and variations within a business to provide beneficial insights and predictions on what a business is to expect, areas to improve, new markets to explore, and customer behaviors to plan for. Predictive analytics is particularly relevant for business managers and consultants who provide guidance and professional counseling for businesses in diverse niches and stages.
At Infomineo, we exceed every client’s expectations by providing reliable, excellent, and unmatched big data analytics services.

Benefits of Big Data Analytics Services
Big data analytics is critical for the growth and expansion of businesses, especially in making consequential decisions. The right decision, defined by valuable insights, can transform and grow a business. With that said, here are some remarkable benefits of big data analytics services for businesses:
Enhanced Decision-making
Big data analytics provides decision-makers with real-time and previous data, which allows them to make informed decisions, initiate radical growth, and boost the Return on Investments (ROI) of their businesses. With predictive analytics evaluating previous sales data, market trends, and economic/political indicators, business decision-makers can effectively understand and predict demand. Thus, they can optimize supply chains by planning inventories and maintaining a balance between stock levels and demand. Overall, a business can better serve its clients, gain their loyalty, and develop solid market expansion strategies.
Cost Efficiency
2023 statistics show that companies that use big data experience experience an average profit increase of 8%. By predicting possible surges in the prices of raw materials, predictive analytics of big data can allow manufacturing companies to stock more for future production and avoid wrong decisions that can lead to significant losses and operational resource waste. Furthermore, businesses would need to implement data warehousing by storing all data in one place, allowing them to save on the costs associated with managing numerous data repositories.
Risk Management
Predictive analytics analyzes historical data, patterns, and trends to identify potential risks and help businesses avert them. An example includes recognizing unstable markets with high failure potential, which can help businesses draft the appropriate strategies to overcome those risks. Predictive analytics can also help in fraud detection, especially in finance and cybersecurity, helping such sectors avoid potential security challenges.
Improved Customer Experience
Descriptive, diagnostic, and predictive analytics can devise new ways to satisfy customers. Using AI and machine learning algorithms, big data analytics can identify customer pain points, their buying habits and preferences, and even what they will buy next. Armed with this information, a business can tailor its marketing campaigns, products, and services to address the dire needs of its customer base and improve their experiences. This can lead to customer acquisition, trust, loyalty, and retention.
Choosing the Right Big Data Analytics Services Provider
Choosing the right big data analytics service provider requires thorough analysis and is critical to benefiting from big data. Here are some factors to consider when searching for the right provider for you.
Level of Specialization
When choosing, consider specialization as a primary criterion for selection. Some big data analytics providers specialize in specific niches like mobile data, social media data analytics, health, and finance, while others may focus on big data in its comprehensive form. Your choice will depend on the kind of data you want to analyze and the insights that you seek to derive.
Expertise and Prior Expertise
Big data is massive and complex, which makes its analytics challenging. A data analytics provider with significant years of experience and a track record of successful projects should be your choice. Below are some important questions you must ask to evaluate the expertise and quality of service of the provider:
- How long have they been in business?
- How many clients have they satisfied?
- What does their feedback section look like?
- How many big brands have they worked with?
- What are the latest technologies they are deploying?
Data Management and Security
Beyond expertise, it is important to inquire whether the providers are in full compliance with data management and security policies. What are their strategies to prevent data breaches or bounce back when faced with security challenges? Are they operating in line with regulatory bodies and their policies in the data industry? These considerations help you choose the best hands for the job and a fail-safe data analytics provider.
Technology Stack and Resources
The technology and resources a provider uses for big data analytics determine the quality of results they can deliver. It is important to ensure they use the latest tools and data fit for your specific industry to guarantee real-time data sourcing and efficiency. Additionally, understanding their system integration process is crucial for an efficient transition.
Collaboration Models
Transparent communication and flexibility in responding to emerging challenges or changing project needs are key considerations when choosing a big data analytics provider. This would allow you to build a trust-based relationship, ensure scope and expectations alignment, have visibility on project progress, and provide feedback as needed.
Skip the stress of looking for a reliable big data analytics services provider that can specifically address your business.
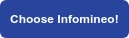
Implementing Big Data Analytics in Your Organization
Implementing big data analytics in your organization can drive growth, boost profit potential, and keep your business ahead of the competition.Here are 5 integration strategies to implement big data in your business:.
1. Evaluate Your Data Needs
Before any implementation, data needs to be evaluated against your needs by answering a few questions, including:
- What are the challenges that big data analytics services can solve in my business?
- What are the profitable opportunities that big data can uncover for my business?
- How much data does my business need to grow?
- What type of data would my business require?
- What are the goals and objectives I seek to achieve with big data analytics services?
Answering these questions will help you find the right big data analytics service provider, the type of analysis you need, your data sources, and other information you may require while integrating big data.
2. Select a Big Data Platform
Next, you need to choose a data platform to integrate into your existing IT infrastructure; it can be cloud, on-premise, or both (hybrid). This platform refers to the data infrastructure and software that will manage, process, analyze, and store your data for easy access. Your choice of platform will depend largely on your budget and the data needs you determined in the first step, in terms of room for scalability, compatibility with existing systems, performance, and features of the data platform such as data quality, security, and integration.
3. Choose Agile Implementation
It is paramount to consider agile techniques when implementing big data analytics. Agility in the business context is the ability of a business to instantly respond to changes as they happen without obstructing its day-to-day operations. Hence, using agile techniques will help your business adapt to changes that may arise within the implementation process and stay up to date with trends.
4. Implement Big Data One Step at a Time
Depending on the size of your organization, implementing big data analytics may require a step-by-step approach, beginning with your existing data. Attempting to delve into the process all at once and involving all data sets can be counterproductive, as the results may be too complex, unreliable, and difficult to analyze. Start the implementation process with your existing data sets and gradually work your way up to larger data sets from various sources.
5. Communicate your Data Analysis Results
After gaining insights from the data analytics process, it is necessary to communicate and tailor them to your target audience, whether they are customers, executives, or others. You can deploy formats such as graphs, reports, pictograms, and charts for transparent data visualization as well as summaries, action points, and recommendations for narratives and data storytelling. Depending on your target audience, you can focus on notable findings, patterns, correlations, trends, or habits.
6. Evaluate and Track your Data Strategy
Finally, it is necessary to evaluate your data strategy by weighing its performance in the implementation process and tracking the results you get. This can be done by evaluating your Key Performance Indicator (KPI), Return on Investment (ROI), and Net Promoter Score (NPS) to improve your existing data strategy or map out a new one. You will also identify loopholes to cover and opportunities to leverage while ensuring that you reach your goals and objectives for implementing big data analytics in your business.
Industry-specific Applications of Big Data Analytics
Big data analytics services have grown across different industries globally. Some applications include the following:
Banking
In the banking sector, big data analytics is a powerful resource for tracking the spending habits of credit card holders, detecting unusual patterns, and flagging suspicious activities that may lead to fraud. Banks also deploy it to keep an eye on employee performance, measure and report KPIs, and manage operational activities and risks.
Healthcare
Big data analytics services are transforming healthcare service delivery globally. They are behind the prediction of global epidemic outbreaks, research speeds, electronic health records, and early detection of disease symptoms to avoid contamination. Some hospitals use big data analytics to provide evidence-based medicine by analyzing data from millions of patients through smartphone apps, instead of relying solely on medical and lab tests for each patient.
Manufacturing
Predicting product failure and maintenance costs, reducing production time, and assessing the best price point for businesses and customers are some of the many benefits of deploying big data analytics services in the manufacturing industry.
Retail
Data in the retail sector is sourced from Point of Sale (POS) scanners, Radio Frequency Infrared Devices (RFIDs), loyalty cards, inventories, and specific demographics. They are then used to market products, improve customer experience, retain customers, and even reduce fraud.
Government
Governments are perhaps the most prominent big data collectors. For instance, the Federal Bureau of Investigation (FBI) uses it to monitor various markets and identify criminal business transactions. Similarly, the Federal Housing Authority analyzes it to predict mortgage repayment rates and identify defaulters. Government agencies such as the Internal Revenue Service (IRS) also depend on big data analytics services to detect and prevent tax fraud.
Challenges and Solutions in Big Data Analytics Services
Big data analytics services can bring positive change to a business’s profit landscape, but they also present challenges. The massive volume, ultra-fast speed, expansive variety, and sheer complexity of big data make it difficult to integrate into existing operational systems. Some challenges of big data and how to address them include:
Data Security
With big data, there is a heightened risk of data security challenges and privacy violations, as cybercriminals tend to focus on sensitive information stored in large volumes. They hack into data banks, costing organizations time, money, and reputation. Deploying artificial intelligence solutions can help guard data sets, maintain round-the-clock surveillance, and raise an alarm on discovering any suspicious activities. Businesses can also employ cybersecurity experts to maintain surveillance of their data warehouses and implement security protocols that will control access rights, encrypt sensitive data with secure login credentials, and integrate on-premise data warehouses.
Data Quality
Data quality refers to the completeness and accuracy of data, which can be difficult to attain with big data, leading to wrong decisions. Organizations can overcome data quality issues by using automated tools that sweep through data to search for errors, inconsistencies, duplicates, and other irregularities.
Communication and Alignment
When dealing with big data, various complex data sets are in the mix, which may lead to communication challenges between big data analytics teams. Some of them include the following:
- The use of technical jargon by the Data Analytics team that other non-technical teams involved may not understand
- Poor communication, feedback, and evaluation across teams
- Lack of alignment on the goals, scope, and objectives of the big data analytics implementation program
Potential solutions to overcome these challenges could be the following:
- Simplifying complex terms and explaining acronyms
- Maintaining open communication and accurate feedback across teams
- Investing in high-end communication tools
- Onboarding all involved parties to the goals, dynamics, objectives, and scope of the big data implementation program
Big data is here to stay and can only improve with time. The increase in web traffic and web-based applications only means that big data will continue to rise in relevance.
Data Analysis
Mistakes and misinterpretations are not uncommon when it comes to big data and can hinder successful implementation. The sheer volume and high speed of big data, coupled with the lack of trained and experienced data professionals, is an ongoing challenge for companies. A deep understanding of big data and extensive experience can help mitigate this challenge. Based on their budget, organizations can invest in a big data specialist to train their staff, either by joining the team or working on a contractual basis.
How Infomineo is Leading the Way in Big Data Analytics with its Distinctive Methodology
Infomineo specializes in data engineering, extraction, analysis, and visual representation. We gather information from various online sources, including Google searches, social media platforms, and websites, and organize the data based on distinct characteristics. Our focus is on data correlation to detect patterns, which we present through interactive interfaces such as dashboards using tools like Power BI, Tableau, and advanced Excel charts.
Our strength lies not only in analyzing large datasets but also in merging it with our business acumen. This allows us to provide customized insights that align with each client’s strategic goals. We enhance businesses’ internal data by complementing and benchmarking it against external contexts. Our Data Analytics team comprises professionals who are well-versed in diverse industries, ensuring that their solutions harness the power of data and align strategically with industry-specific challenges.
Frequently Asked Questions (FAQs)
How can I tell if my business demands big data analytics services?
You can tell if your business will require big data analytics services by evaluating your current data needs and the size and objectives of your business. This evaluation will reveal how much data you have, how much more you require, and the type of analytics you need, among other relevant information.
What are the applications of big data analytic services?
Big data applications cut across various niches and industries, ranging from the healthcare and baking sectors to the education, retail, manufacturing, and public sectors.
How can I choose the right big data services provider?
You can make the right choice by conducting your due diligence or background check on the provider. Look for reviews and testimonials, check their client base, identify their technological resources, and find out if they can address your business’s specific needs.
What are the main challenges of big data analytics?
The principal challenges of big data analytics are the 3Vs – Volume, Velocity (Speed), and Variety. Analyzing enormous volumes of data coming at high speeds and in their varieties is a complex challenge.
How does big data processing differ from traditional data processing?
Traditional data processing only handles structured or well-defined data and is limited in volume and variety, while big data processing handles both structured and unstructured data in large volumes and varieties.
Conclusion
Data is a fundamental resource for every business, whereas big data is a transformational tool for growth. Deploying big data analytics services gives businesses access to a large pool of data sets in their variety that can identify patterns and relationships, learn customer habits via machine learning, and predict the future with AI. These services can help a business stay on top of trends, grow exponentially, satisfy customers, stay ahead of the competition, and avoid surprises via predictive analytics.