A Comprehensive Guide to Descriptive, Predictive and Prescriptive Analytics
A Comprehensive Guide to Descriptive, Predictive and Prescriptive Analytics
Data is everywhere, shaping decisions in businesses, industries, and our daily lives. The global generation of data is increasing at an unprecedented rate, creating both challenges and opportunities for organizations eager to harness this information for more accurate decision-making. Analytics equips these organizations with the essential tools and techniques to extract meaningful insights and facilitate informed actions.
In this comprehensive guide, we will explore three powerful types of analytics: descriptive, predictive, and prescriptive. We will examine the techniques leveraged in each type, including data aggregation, regression analysis, and optimization algorithms. Additionally, we will highlight the diverse applications of analytics in various sectors, such as business, healthcare, finance, and manufacturing.
We will also discuss the advantages and disadvantages of each type of analytics, providing a balanced perspective on their strengths and limitations. Ultimately, this article aims to provide a clear understanding of how these analytical approaches can unlock the true potential of data and drive success across multiple fields.
Descriptive Analytics
Descriptive analytics serves as the foundation of data analysis by examining past data to uncover insights into what has occurred. This approach organizes and summarizes historical information to identify trends, patterns, and key metrics, enabling organizations to better understand their performance and make informed decisions. Techniques such as data aggregation, data mining, data visualization, statistical analysis, and key performance indicators (KPIs), along with their applications across different fields, provide a comprehensive understanding of the importance of descriptive analytics.
Techniques of Descriptive Analytics
Data analytics employs various techniques to extract valuable insights from the data. Some of these techniques include:
Data Aggregation
Data aggregation is a technique that collects data from various sources, combining it into a single, coherent dataset. This process involves cleaning and organizing the information to streamline analysis. For example, a retail chain may aggregate sales data from all its stores to gain a comprehensive view of its overall performance.
Data Mining
Data mining uses advanced algorithms to uncover hidden patterns and relationships within large datasets that might otherwise go unnoticed. For instance, a bank could use data mining to identify common characteristics among customers who are likely to default on loans.
Data Visualization
Data visualization is the representation of data through charts, graphs, and interactive dashboards, to facilitate pattern identification and comprehension. For example, weather forecasters can use color-coded maps to illustrate temperature changes over time.
Statistical Analysis
Statistical analysis, which can take the form of trend analysis and comparative analysis, is a technique that uses mathematical methods to interpret and draw conclusions from data. Trend analysis tracks data over time to identify upward or downward movements, while comparative analysis looks at differences between groups. For instance, a social media platform might use trend analysis to track user engagement over a few months, and comparative analysis to understand how engagement differs across age groups.
Key Performance Indicators (KPIs)
Key Performance Indicators (KPIs) are specific, quantifiable metrics that organizations use to measure their progress towards strategic objectives. These carefully selected indicators help organizations monitor their performance in various areas such as human resources, marketing, finance, and operations. KPIs provide a clear and concise framework for evaluating the effectiveness of business processes and strategies by focusing on a few crucial measurements. For example, HR departments may track employee turnover rates and training completion percentages, while marketing teams might focus on website traffic and conversion rates.
Applications of Descriptive Analytics
Descriptive analytics is used in various fields, enabling organizations to derive meaningful insights from their data. Below are some key areas where it is making a significant impact:
- Business reporting: Regular reporting on sales, revenue, and other KPIs empower businesses to make accurate decisions. These reports distill complex data into clear summaries, allowing managers to track progress and identify trends. Research shows that most organizations use descriptive analytics for financial reporting, underscoring its vital role in business intelligence.
- Customer segmentation: Companies can group their customer data based on shared characteristics, such as buying habits or demographics to enhance targeted marketing and create personalized experiences. For instance, e-commerce platforms can segment shoppers by purchase frequency and average order value.
- Market analysis: Descriptive analytics helps businesses understand market trends and consumer behavior by revealing patterns in customer preferences, identifying emerging opportunities, and informing product development.
- Operational efficiency: By monitoring business processes, including supply chains, inventories, and employee productivity, businesses can identify bottlenecks, improve efficiency, and reduce costs.
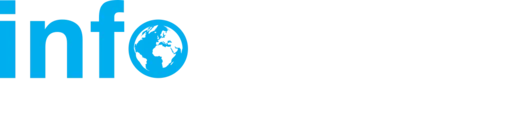
Predictive Analytics
Predictive analytics leverages historical data and applies statistical techniques to make educated guesses about future events. By identifying patterns and trends within past data, predictive analytics enables businesses and organizations to forecast future outcomes and make proactive decisions. It uses techniques such as regression analysis, time series analysis, and data mining. Exploring these methods and their applications across various fields will provide a comprehensive understanding of this powerful data analysis approach.
Techniques for Predictive Analytics
Predictive Analytics employs various sophisticated methods to forecast future outcomes. Some of the key techniques that power these predictions are:
Regression Analysis
This technique explores relationships between variables, quantifying cause-and-effect relationships to understand how one factor can affect another. For example, it can reveal how changes in advertising spend impact sales, or how fluctuations in raw material prices influence production costs in a supply chain.
Time Series Analysis
Time series analysis studies historical data to identify patterns, forecast future outcomes, and better prepare for them. This technique is particularly useful for seasonal predictions, such as retail sales during holidays.
Machine Learning Algorithms
These algorithms make predictive analytics very powerful and reliable. Machine learning algorithms continuously improve their predictions as they learn from new data and can handle complex patterns that humans might overlook. From product recommendations on e-commerce sites to equipment failure predictions in factories, these algorithms are revolutionizing all industries.
Classification Models
Classification models categorize new data into predefined groups based on patterns learned from historical examples. For instance, a model trained on customer data could predict whether a new customer is likely to respond to a promotional email based on their characteristics. Classification models are a specific type of machine learning algorithm that focus on categorizing data into discrete classes. Other types of machine learning algorithms serve different purposes, such as predicting continuous values or identifying inherent groupings within data.
Data Mining
This process employs statistical algorithms and machine learning techniques to identify significant patterns, correlations, and anomalies within large and complex datasets. Unlike time series analysis, which focuses on data points collected over time, data mining can be applied to various types of data, automatically discovering non-obvious insights that might be overlooked in manual analysis. Its applications in fields such as market basket analysis, fraud detection, and customer segmentation showcase its versatility in predictive analytics.
Applications of Predictive Analytics
Predictive Analytics is transforming various sectors. Some of the key areas where it has a significant impact include:
- Risk Management in the Oil and Gas Sector: This involves identifying and mitigating potential threats, allowing companies to proactively address uncertainties.
- Customer Retention in the Retail Sector: Predictive models help detect early signs of customer dissatisfaction by analyzing purchase patterns and engagement metrics.
- Disease Prevention in the Healthcare Sector: Predictive modeling enables the scanning of patient data, medical histories, and population health trends to anticipate disease outbreaks.
Prescriptive Analytics
Prescriptive analytics is an advanced approach to data analysis that offers targeted recommendations to optimize decision-making. By employing complex algorithms and machine learning techniques, it analyzes various scenarios and constraints to identify the optimal course of action. Understanding the techniques and applications of prescriptive analytics is essential for gaining a comprehensive insight into its capabilities and how it can drive informed decisions across different sectors.
Techniques of Prescriptive Analytics
The techniques of prescriptive analytics are designed to provide actionable insights and recommendations based on complex data analysis. Some of the key methods employed in prescriptive analytics include:
Optimization Algorithms
Optimization algorithms are mathematical techniques that identify the most effective solution to a problem by systematically evaluating a wide range of viable options and constraints. The algorithms analyze various potential network configurations and identify the one that minimizes total costs while ensuring timely delivery to customers. For example, in supply chain management, optimization algorithms can determine the most efficient distribution network by considering factors like transportation costs, warehouse locations, and customer demand.
Simulation Models
Simulation models are computer-based representations of real-world systems that allow decision-makers to evaluate the potential outcomes of various scenarios and strategies without incurring the risks or costs associated with real-world implementation. They enable organizations to proactively assess the impact of different decisions and external factors on their operations. By manipulating input variables and observing the resulting changes in the model’s behavior, decision-makers can gain valuable insights into the complex interactions and dependencies within their systems.
Decision Analysis
Decision analysis is a structured approach to evaluating and comparing alternative courses of action when faced with complex business decisions. It involves defining clear objectives, identifying potential options, and assessing each option based on relevant criteria and their relative importance. Unlike simulation models, which focus on understanding the dynamic behavior of a system, decision analysis emphasizes the systematic evaluation of discrete decision options to identify the most advantageous path forward. It is particularly useful when facing high-stakes decisions with multiple conflicting objectives.
Machine Learning
Machine learning algorithms improve their performance over time by learning from new data. They can spot patterns humans might miss and make increasingly accurate predictions. In prescriptive analytics, machine learning algorithms go beyond predicting future outcomes by suggesting optimal actions based on historical and real-time data. This allows organizations to make dynamic and data-driven decisions.
Scenario Analysis
Scenario analysis is a method that evaluates the potential outcomes of alternative future events or decisions by considering a range of situations. It examines the impact of specific, discrete scenarios on an organization’s objectives. By exploring different “what-if” situations, scenario analysis helps decision-makers develop contingency plans and make more informed strategic choices.
Applications of Prescriptive Analytics
Prescriptive analytics is widely used in various industries. Some of its applications include the following:
- Manufacturing: Factories use prescriptive analytics to fine-tune operations. The system might suggest adjusting machine speeds to boost output or recommend maintenance before breakdowns occur. It can also balance production schedules with storage capacity, which ensures smooth operations.
- Hospitality: Airlines and hotels can harness prescriptive analytics to set prices that maximize profits. The system analyzes demand patterns, competitor pricing, and weather forecasts to suggest optimal rates. It might recommend lowering prices to fill empty seats or rooms or raising them during peak times.
- Healthcare: Prescriptive analytics help medical experts recommend personalized treatment plans. It considers a patient’s medical history, genetic factors, and lifestyle to suggest the most effective therapies and the best timing for them.
- Finance: Investment firms use prescriptive analytics to build smarter portfolios and navigate volatile markets. By analyzing market data, risk factors, and investor preferences, it recommends optimal asset allocations and suggests strategic adjustments based on global events and individual risk tolerance.
Advantages and Disadvantages of Descriptive, Predictive, and Prescriptive Analytics
Each type of analytics offers distinct advantages that can provide valuable insights and support data-driven decision-making. However, it is equally important to recognize their limitations and potential drawbacks. By thoughtfully evaluating both the strengths and weaknesses of these analytical techniques, organizations can make informed decisions about which approach to leverage in specific scenarios.
TYPE OF ANALYTICS | ADVANTAGES | DISADVANTAGES |
---|---|---|
Descriptive |
Uncovers hidden patterns and new concepts for further research Offers broader insights compared to typical quantitative methods Requires minimal statistical expertise to implement Seamlessly integrates into routine business processes |
Provides data summaries without explaining underlying causes or predicting future trends Is confined to basic analyses involving few variables |
Predictive |
Boosts efficiency via precise inventory forecasts, streamlined supply chains, and proactive maintenance Strengthens fraud detection by identifying subtle patterns and anomalies Mitigates risks in finance through improved candidate screening and in IT by flagging potential security threats Elevates customer service by providing deeper insights into customer preferences for tailored recommendations |
Presents inherent uncertainty due to probability-based predictions, potential data limitations, and unforeseen factors Demands substantial resources and specialized expertise Requires constant data set updates to maintain relevance Overly complex or customized models may yield inaccurate predictions when applied to new data sets |
Prescriptive |
Maps out multiple action paths with predicted outcomes for each scenario Leverages advanced modeling and algorithms to surpass human speed and accuracy, minimizing error risk |
Demands vast data sets for meaningful results, sometimes exceeding available resources Necessitates substantial computing power and oversight from specialized machine learning experts, driving up costs and time investment Vulnerable to data quality issues, potentially leading to skewed recommendations Risks inappropriate actions in automated decision-making contexts Typically involves a lengthy implementation process |
Infomineo: Leading the Way in Descriptive, Predictive, and Prescriptive Analytics
Infomineo specializes in descriptive, predictive, and prescriptive analytics, guiding our clients in selecting the most suitable type of analytics based on their organizational objectives and data infrastructure.
Clients with both business and technical expertise can reach out to us with specific analytics needs, and we develop tailored and effective solutions to address them. By gathering and organizing information from various internal and online sources and leveraging advanced techniques to analyze large datasets, we uncover patterns and generate predictions.
Our customized solutions cater to diverse industries, ensuring that insights align with our clients’ strategic goals, such as matching supply and demand through predictive analytics. Our skilled professionals deliver insights through interactive dashboards using tools like Power BI and Tableau, seamlessly integrating with clients’ teams.
Frequently Asked Questions (FAQs)
What is the main difference between the three types of analytics?
Descriptive analytics looks at past data to understand what happened, while predictive analytics uses that historical data to forecast future trends. Descriptive analytics tells you “what occurred,” whereas predictive analytics suggests “what might occur next” based on patterns in the data. Prescriptive analytics takes it a step further by not only predicting future outcomes but also recommending specific actions to optimize results.
How does prescriptive analytics improve decision-making?
Prescriptive analytics goes beyond prediction by recommending specific actions. It analyzes various scenarios and their potential outcomes, then suggests the best course of action to achieve the desired results. This helps businesses make data-driven decisions with more confidence and precision.
How can businesses benefit from data analytics?
Businesses of all sizes can use analytics. Small businesses can start with descriptive analytics to understand their current performance, then gradually adopt predictive and prescriptive methods as they grow. Larger enterprises can leverage advanced analytics across various departments and industries to optimize processes and improve decision-making.
What are the key techniques used in descriptive, predictive, and prescriptive analytics?
Descriptive analytics employs techniques like data aggregation, data mining, data visualization, statistical analysis, and key performance indicators (KPIs). Predictive analytics utilizes regression analysis, time series analysis, machine learning algorithms, classification models, and data mining. Prescriptive analytics leverages optimization algorithms, simulation models, decision analysis, machine learning, and scenario analysis to provide data-driven recommendations for decision-making.
How reliable are the predictions made by predictive analytics?
Predictive analytics’ reliability depends on data quality and model accuracy. While it can provide valuable insights, it is fallible. Predictions should be used as guides alongside human judgment, and models should be regularly updated with new data to maintain accuracy.
To Sum Up
Data analytics has become a crucial tool for businesses looking to improve their decision-making processes. Descriptive analytics, which employs techniques like data aggregation, data mining, data visualization, statistical analysis, and KPIs, provides insights into past performance. Predictive analytics, utilizing regression analysis, time series analysis, machine learning algorithms, classification models, and data mining, forecasts future trends which enables organizations to prepare for what lies ahead.
Prescriptive analytics leverages optimization algorithms, simulation models, decision analysis, machine learning, and scenario analysis to recommend specific actions that optimize decision-making and achieve the desired outcomes.
All three types of data analytics have multiple cross-industry applications, such as business, finance, healthcare, manufacturing, retail, telecommunications, energy, and transportation. The true power of data analytics lies in combining these methods to gain a holistic view of an organization’s operations and make informed, data-driven decisions. As data continues to grow in volume and importance, mastering these analytics techniques will be essential for staying competitive and thriving in an increasingly data-centric world.