AI-Powered Analytics vs. Traditional Data Analysis: Which Offers Better Insights for Consultancy Firms?
AI-Powered Analytics vs. Traditional Data Analysis: Which Offers Better Insights for Consultancy Firms?
Data analysis plays a crucial role in helping consultancy firms make informed decisions and provide valuable insights to their clients. The common approaches consultancy firms use for data analysis include traditional data analysis and AI-powered analytics. Traditional data analysis involves using conventional statistical methods and human expertise to examine data, while AI-powered analytics leverages machine learning algorithms and artificial intelligence to discover patterns and insights.
As consultancy firms strive to stay competitive and deliver the best possible solutions to their clients, it is essential to understand the differences between these two approaches and determine which one offers better insights. This article will explore the strengths and weaknesses of AI-powered analytics and traditional data analysis and by doing that help consultancy firms make informed decisions about which approach to adopt for their data analysis needs.
Understanding Traditional Data Analysis
Traditional data analysis is the process of examining, cleaning, transforming, and modeling data using conventional statistical methods and human expertise to discover useful information, draw conclusions, and support decision-making. It relies on manual analysis techniques and does not involve advanced artificial intelligence or machine learning algorithms.
Methods and techniques used in traditional data analysis include:
- Descriptive statistics: This involves summarizing and describing the main features of a dataset like measures of central tendency (mean, median, mode) and measures of dispersion (range, variance, standard deviation).
- Exploratory data analysis (EDA): EDA is used to understand the characteristics of a dataset, identify patterns, and detect anomalies through visual and statistical techniques like histograms, box plots, and scatter plots.
- Hypothesis testing: This method involves formulating a null hypothesis and an alternative hypothesis then using statistical tests (e.g., t-tests, chi-square tests, ANOVA) to determine whether the null hypothesis can be rejected in favor of the alternative hypothesis.
- Regression analysis: Regression is used to examine the relationship between a dependent variable and one or more independent variables. It helps predict future values of the dependent variable based on the independent variables.
- Time series analysis: This technique is used to analyze data collected over time, identifying trends, seasonality, and other patterns. It is useful for forecasting future values based on historical data.
Strengths of Traditional Data Analysis
Traditional data analysis has several strengths that have made it a reliable approach for consultancy firms. These strengths include:
- Interpretability: Traditional data analysis methods are often easier to interpret and understand compared to complex AI-powered analytics. This clarity allows decision-makers to grasp the insights more readily.
- Transparency: The steps involved in traditional data analysis are typically more transparent, allowing analysts to explain their process and reasoning behind the conclusions drawn from the data.
- Requires less data: Traditional methods can often work effectively with smaller datasets which is advantageous when data is limited or expensive to acquire.
- Established best practices: Traditional data analysis benefits from well-established best practices and proven methodologies thus providing a solid foundation for analysis and decision-making.
- Accessibility: Many traditional data analysis techniques can be performed using widely available software tools and do not require specialized hardware or extensive computing resources.
Limitations of Traditional Data Analysis
Despite its strengths, traditional data analysis also has several limitations that can hinder its effectiveness in certain consultancy firm operations. These limitations include:
- Scalability: Traditional methods can struggle to handle extremely large datasets or complex data structures leading to longer processing times and reduced efficiency.
- Limited insights: Traditional techniques may miss hidden patterns or relationships in the data that more advanced AI-powered analytics can uncover.
- Manual effort: Traditional data analysis often requires significant manual effort and expertise which can be time-consuming and prone to human error.
- Bias: Human analysts may inadvertently introduce bias into the analysis based on their own experiences, assumptions, or preconceptions.
- Adaptability: Traditional methods may not be as adaptable to changing data landscapes or real-time analysis requirements compared to AI-powered approaches.
Introduction to AI-Powered Analytics
AI-powered analytics is the process of using artificial intelligence and machine learning algorithms to analyze data, uncover insights, and make predictions. This approach leverages the power of AI to process vast amounts of data quickly and accurately, enabling consultancy firms to gain a competitive edge and provide more value to their clients compared to traditional data analysis methods. A report by Gartner predicts that by 2028, 75% of organizations will shift from piloting to operationalizing AI, driving a 5x increase in streaming data and analytics infrastructures.
Key technologies in AI-powered analytics include:
- Machine Learning (ML): ML algorithms allow systems to learn from data and improve their performance without being explicitly programmed. In consultancy firms, ML can be used to identify patterns, predict outcomes, and optimize processes thus providing insights that may be missed by traditional data analysis techniques.
- Deep Learning (DL): DL is a subset of ML that uses artificial neural networks to model and solve complex problems. DL is particularly useful for analyzing unstructured data like images, videos, and text, which is often challenging for traditional data analysis methods. Consultants can use DL to extract valuable insights from diverse data sources.
- Natural Language Processing (NLP): NLP enables computers to understand, interpret, and generate human language. In consultancy firms, NLP can be used to analyze customer feedback, social media posts and other text-based data sources and so provide insights into customer sentiment and preferences.
- Computer Vision: Computer vision allows computers to interpret and understand visual information from the world around them. Consultants can use computer vision to analyze images and videos to extract valuable insights and inform decision-making.
- Big Data Analytics: AI-powered analytics is well-suited to handle big data, which refers to datasets that are too large and complex for traditional data analysis tools. Thanks to big data analytics, consultancy firms can uncover hidden patterns and correlations that may not be apparent using traditional methods.
Benefits of AI-Powered Analytics
AI-powered analytics offers numerous benefits to consultancy firms by enabling them to process large volumes of data efficiently and extract valuable insights to support data-driven decision-making. According to the 2024 PwC Global CEO Survey, 70% of business leaders believe that generative AI will significantly change the way their business creates, delivers and captures value. Some of the key benefits include:
- Improved accuracy: AI algorithms can analyze data with a higher degree of accuracy than human analysts hence reducing the risk of errors and ensuring more reliable insights.
- Increased efficiency: AI-powered analytics can process vast amounts of data quickly which saves time and resources compared to manual analysis methods.
- AI scalability: As data volumes grow, AI-powered analytics can easily scale to accommodate larger datasets ensuring that consultancy firms can continue to derive insights from their data.
- Real-time insights: AI algorithms can analyze data in real-time. Consultancy firms can leverage this to make timely decisions based on the most up-to-date information.
- Predictive capabilities: AI-powered analytics can identify patterns and trends in data allowing consultancy firms to make predictive insights and anticipate future outcomes.
Challenges of AI-Powered Analytics
Even though there are numerous benefits of AI-powered analytics, consultancy firms must also be aware of the challenges associated with implementing and using these technologies. Some of the key challenges include:
- Data quality: AI algorithms require high-quality, clean, and structured data to function effectively. Ensuring data quality can be a significant challenge especially when dealing with large, diverse datasets.
- Interpretability: Some AI models, particularly those based on deep learning, can be difficult to interpret making it challenging for consultants to explain the reasoning behind the insights generated.
- Skill requirements: Implementing and using AI-powered analytics requires specialized skills in data science, machine learning, and AI. Consultancy firms may need to invest in training or hiring personnel with these skills.
- Integration with existing systems: Integrating AI-powered analytics with existing data infrastructure and systems can be complex and time-consuming.
Consultancy firms must carefully consider these challenges when implementing AI-powered analytics to ensure they can effectively leverage the technology to drive data-driven decision-making while mitigating potential risks and drawbacks.
Comparison of AI-Powered Analytics vs. Traditional Data Analysis
When considering the best approach to data analysis for their organization, consultancy firms must carefully weigh the differences between AI-powered analytics and traditional data analysis. A thorough comparison of these two methods across key aspects will enable decision-makers to select the approach that aligns with their specific needs, resources, and goals.
Aspect | AI-Powered Analytics | Traditional Data Analysis |
---|---|---|
Data handling | Excels at processing large, complex datasets | Limited by data size and complexity |
Speed and efficiency | Analyzes data quickly and efficiently | Time-consuming and resource-intensive |
Insight discovery | Uncovers hidden patterns and relationships | May miss subtle insights |
Skill requirements | Requires specialized AI and data science skills | Relies on traditional statistical and analytical skills |
Interpretability | Some models can be difficult to interpret | Results are generally easier to interpret and explain |
Adaptability to change | Adapts quickly to new data and evolving requirements | Less flexible and adaptable to rapid changes |
AI-Powered Analytics for Consultancy Firms
AI-powered analytics is a game-changer for consultancy firms. It offers unprecedented opportunities to extract valuable insights from vast datasets and deliver tailored solutions to clients. Thanks to machine learning algorithms and advanced analytics techniques, consultants can uncover hidden patterns, predict future trends, and optimize decision-making processes. This revolutionary approach to data analysis is not only transforming consultancy operations but also redefining the value proposition of consultancy services. Firms are able to tackle their clients’ most pressing challenges with unparalleled precision and effectiveness.
The applications of AI-powered analytics in consultancy firms are diverse and far-reaching:
- Proactive risk management: AI algorithms can continuously monitor market trends, financial data, and other key indicators, empowering consultants to provide clients with real-time risk assessments and develop strategies to mitigate potential threats and capitalize on emerging opportunities.
- Enhanced fraud detection: Sophisticated AI-driven anomaly detection and pattern recognition techniques enable consultancy firms to identify suspicious activities with remarkable accuracy which helps clients prevent financial losses and protect their reputation.
- Personalized customer targeting: AI-powered segmentation and profiling techniques allow consultants to analyze vast customer datasets and help them identify unique preferences and behaviors. As a result, businesses can leverage digital personalization at scale to ensure their marketing campaigns and sales strategies resonates with individual customers.
- Optimized equipment maintenance: Predictive analytics, fueled by AI, empowers consultancy firms to help clients optimize maintenance schedules for critical assets. This reduces downtime, minimizes repair costs, and extends equipment lifespans.
- Dynamic supply chain optimization: By continuously analyzing real-time supply chain data using AI algorithms, consultants can help their clients identify and eliminate inefficiencies, anticipate and mitigate potential disruptions.
Advantages for Consultancy Firms
Embracing AI-powered analytics offers consultancy firms a range of compelling advantages:
- Enhanced decision-making: AI-driven insights provide consultants with the real-time, actionable intelligence needed to help clients make informed decisions quickly and confidently.
- Improved efficiency and scalability: Automating and streamlining data analysis tasks through AI-powered analytics allows consultancy firms to deliver high-quality results more efficiently.
- Competitive differentiation: As AI-powered analytics becomes increasingly crucial for success in the consulting industry, firms that excel in leveraging these technologies will stand out from competitors and attract clients seeking innovative, data-driven solutions.
- Increased client satisfaction and loyalty: Consistently delivering impactful insights that drive measurable results helps consultancy firms build stronger, more trusting relationships with clients.
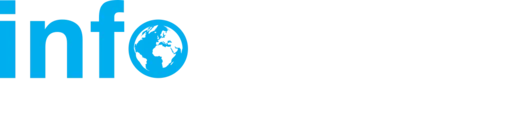
Traditional Data Analysis for Consultancy Firms
Despite the rapid rise of AI-powered analytics, traditional data analysis techniques remain indispensable tools in the consultant’s toolkit. These time-tested methods provide a solid foundation for understanding complex business problems, identifying key drivers of performance, and developing evidence-based recommendations. While AI may grab headlines, the tried-and-tested approaches of traditional data analysis continue to deliver reliable insights that help consultancy firms guide their clients to success in an increasingly data-driven world.
Traditional data analysis finds wide application across various consultancy engagements:
- Market sizing and segmentation: Consultants use statistical techniques like cluster analysis and factor analysis to identify distinct customer segments, estimate market potential, and develop targeted growth strategies.
- Pricing optimization: By analyzing historical sales data, price elasticity, and competitor pricing, consultants help clients optimize their pricing strategies to maximize revenue and profitability.
- Performance benchmarking: Consultants rely on descriptive statistics and data visualization to compare a client’s key performance indicators against industry benchmarks. This helps identify areas for improvement and best practices to emulate.
- Customer lifetime value analysis: Using cohort analysis and predictive modeling, consultants help clients understand the long-term value of their customers.
- Cost-benefit analysis: Consultants apply financial modeling and scenario analysis to evaluate the potential costs and benefits of different business decisions such as investments in new technology or expansion into new markets.
Advantages for Consultancy Firms
Traditional data analysis offers several unique advantages for consultancy firms:
- Interpretability: Traditional techniques produce results that are easier to interpret and communicate to clients.
- Robustness: Well-established statistical methods are less prone to overfitting and more resilient to noisy or incomplete data.
- Skill availability: The widespread availability of professionals trained in traditional data analysis makes it easier for consultancy firms to build and maintain their analytical capabilities.
- Regulatory compliance: In heavily regulated industries, the transparency and explainability of traditional techniques may be preferred or required by authorities.
- Cost-effectiveness: For smaller engagements or clients with limited budgets, traditional data analysis can offer a more affordable alternative to AI-powered solutions while still delivering valuable insights.
Key Factors to Consider in Choosing Between AI-Powered Analytics and Traditional Data Analysis
When deciding between AI-powered analytics and traditional data analysis, consultancy firms must carefully evaluate several key factors to ensure they select the approach that best aligns with their clients’ needs and delivers the most value.
- Project scope and complexity: The nature and scale of the project should be a primary consideration. AI-powered analytics is often better suited for large, complex projects involving vast amounts of data and multiple variables, while traditional data analysis may be more appropriate for smaller, more focused engagements.
- Budget constraints and ROI considerations: The cost of implementing and maintaining AI-powered analytics solutions can be substantial, including investments in technology, infrastructure, and specialized talent. Consultancy firms must weigh these costs against the potential return on investment and consider whether traditional data analysis techniques could deliver similar results at a lower cost.
- Availability of data and infrastructure: AI-powered analytics requires access to large volumes of high-quality, structured data and the necessary computing infrastructure to process and store it efficiently. If clients lack the requisite data or infrastructure, traditional data analysis may be a more practical choice.
- Required expertise and skills: Implementing and applying AI-powered analytics demands specialized skills in data science, machine learning, and software engineering. Consultancy firms must assess whether they have the right talent in-house or can attract and retain professionals with these sought-after skills. Traditional data analysis, while still requiring analytical expertise, may be more accessible given the broader pool of professionals with relevant backgrounds.
- Long-term vs. short-term goals: Consultancy firms should consider their clients’ long-term and short-term objectives when choosing between AI-powered analytics and traditional data analysis. AI-powered solutions may require more upfront investment but can offer significant long-term advantages in terms of scalability and adaptability. Traditional data analysis may be better suited for short-term projects with well-defined goals and limited scope.
Case Studies
AI-powered analytics and traditional data analysis have transformed how consultancy firms operate, enabling them to derive valuable insights and drive better decision-making. Here are real-world examples of companies leveraging these approaches to achieve tangible results.
Examples Of Consultancy Firms Using AI-Powered Analytics
- Amazon: The e-commerce titan harnesses data science to create personalized shopping experiences. By analyzing customer browsing and purchasing patterns, Amazon’s algorithms generate tailored product recommendations and so enhance customer satisfaction and drive sales growth.
- IBM Watson Health: IBM’s AI-powered platform revolutionizes healthcare by offering personalized diagnostic and treatment recommendations. Leveraging natural language processing, Watson analyzes vast medical literature and patient records which empowers doctors to make more accurate and informed decisions.
- PathAI: This innovative company applies machine learning algorithms to assist pathologists in delivering more precise disease diagnoses. PathAI’s system detects patterns and anomalies that may elude human observation by analyzing digital pathology images. This improves diagnostic accuracy and patient outcomes.
Examples Of Consultancy Firms Using Traditional Data Analysis
- Siemens: The industrial powerhouse employs predictive maintenance through data science. By monitoring and analyzing data from manufacturing equipment, Siemens’ machine learning algorithms identify wear and tear patterns. This enables precise scheduling of maintenance activities to minimize downtime and optimize resource utilization.
- PayPal: The online payment pioneer leverages advanced data science techniques to detect and prevent fraud in real-time. Through analyzing transaction data, user behavior, and other relevant variables, PayPal’s system identifies suspicious activities. This goes a long way in safeguarding users’ financial information and maintaining trust in the platform.
- John Deere: The agricultural machinery leader utilizes data science to forecast crop yields. John Deere provides farmers with actionable insights by processing data from equipment sensors, weather patterns, and soil conditions. This allows them to optimize planting and harvesting schedules, maximizing crop productivity while minimizing resource waste.
Comparative Analysis of Results and Lessons Learned
Amazon’s data-driven approach has yielded impressive results, reducing customer service response times by 40% and increasing average order value by 29% through targeted product recommendations. IBM Watson Health has demonstrated a 15% improvement in cancer diagnosis accuracy when assisting oncologists in analyzing complex medical data. Similarly, PathAI’s AI-powered pathology platform has achieved a 25% increase in diagnostic accuracy for challenging cases like rare diseases or cancer subtypes.
Siemens has reported a remarkable 20% reduction in unplanned downtime across its global manufacturing facilities since implementing predictive maintenance solutions. PayPal’s real-time fraud detection system boasts a 99.9% accuracy rate in identifying and blocking fraudulent transactions, minimizing financial losses. John Deere’s predictive analytics have reduced the need for chemical inputs by 25%, resulting in cost savings and environmental benefits, while farmers using their crop prediction system have seen an average 15% increase in yields.
These case studies highlight the importance of choosing the right approach based on specific business needs and data characteristics. Companies faced challenges such as data quality, integration with existing systems, and talent acquisition. However, by investing in the necessary infrastructure, skills, and processes, they were able to overcome these hurdles and achieve measurable success.
Tools and Technologies for AI-Powered Analytics and Traditional Data Analysis
Selecting the right tools and technologies is crucial for consultancy firms looking to deliver high-quality data analysis and insights. It is also important to know how these technologies fit into different analytical approaches.
The popular AI analytics tools being used in the consultancy industry include:
- TensorFlow: Developed by Google, this is an open-source library for machine learning and deep learning widely used for building and deploying AI models.
- PyTorch: An open-source machine learning library based on Torch that is primarily used for computer vision and natural language processing applications.
- H2O.ai: A platform offering a suite of tools for building, deploying, and maintaining AI and machine learning models with a focus on enterprise scalability and ease of use.
- DataRobot: An automated machine learning platform that simplifies the development and deployment of AI models which enables users to quickly build and implement predictive models.
On the other hand, some of the traditional data analysis tools common in consultancy operations are:
- Microsoft Excel: A widely used spreadsheet application that offers a range of built-in functions and tools for data analysis, visualization, and reporting.
- Tableau: A powerful data visualization and business intelligence platform that enables users to connect, analyze, and share data insights across an organization.
- SAS: A comprehensive suite of tools for data management, advanced analytics, and predictive modeling, widely used in various industries.
- R: An open-source programming language and environment for statistical computing and graphics. Widely used in academia and data analysis.
- IBM SPSS: A statistical software package that offers a range of tools for data analysis, predictive modeling, and data visualization, with a focus on ease of use and accessibility for non-technical users.
Comparison of How These Tools Fit into Different Approaches
AI-powered analytics tools like TensorFlow, PyTorch, and H2O.ai are designed to support the development and deployment of machine learning and deep learning models. These tools are typically used by data scientists and machine learning engineers who have specialized expertise in AI and are working on complex, large-scale projects that require advanced analytical capabilities.
On the other hand, traditional data analysis tools (like Excel, Tableau, and SAS) are more accessible to a broader range of users, including business analysts, managers, and executives. These tools are well-suited for smaller-scale projects and data visualization tasks that don’t require the same level of computational power and complexity as AI-powered approaches.
Some tools, like R, can bridge the gap between traditional data analysis and AI-powered analytics. R offers a wide range of packages and libraries for statistical analysis, data visualization, and machine learning. This makes it a versatile tool for both traditional and AI-powered approaches.
Ultimately, the choice of tools will depend on the specific needs and goals of the project as well as the skills and expertise of the team. Consultancy firms may need to maintain a diverse toolkit that includes both AI-powered and traditional data analysis tools to meet the varying requirements of their clients and deliver the most value across a range of engagements.
Future Trends in Data Analysis for Consultancy Firms
The consulting industry faces a transformative period as artificial intelligence (AI) becomes increasingly integral to data analysis and decision-making processes. Two significant trends are shaping the future of data analysis in consulting: the growing influence of AI in decision-making and the integration of AI with traditional data analysis methods. In fact, a 2022 McKinsey survey found that 63% of respondents report revenue increases from AI adoption in the business units where their companies use AI in decision making, up from 22% in 2020.
AI’s Expanding Role in Decision-Making
AI is no longer a futuristic concept but a present-day necessity for consultancy firms seeking to maintain a competitive edge. Firms that effectively implement AI tools can process vast amounts of data and generate predictive insights that drive better decision-making. However, the successful implementation of AI in decision-making requires firms to invest not only in technology but also in developing a deep understanding of AI’s potential and limitations.
Integration of AI With Traditional Methods
While AI is transformative, it is not a substitute for traditional data analysis methods. The most successful consultancy firms will be those that effectively integrate AI with traditional approaches to create a hybrid data analysis model. This amalgamation of techniques allows firms to deliver insights that are data-driven and supported by robust statistical principles. To achieve this, firms must cultivate a collaborative environment that encourages knowledge sharing between AI experts and traditional analysts. Doing so ensures the strengths of each approach are optimally utilized.
Predictions For the Future of Data Analysis in Consulting
Several key trends are poised to shape the future of data analysis in the consulting industry:
- Real-time analytics: The demand for real-time insights will surge as clients seek to remain agile in the face of rapidly evolving market conditions. Consultancy firms must prioritize the development of real-time analytics capabilities to meet this need.
- Cloud-based platforms: The adoption of cloud-based analytics platforms will accelerate, providing firms with the scalability, flexibility, and access to advanced tools necessary to support real-time analytics and hybrid data analysis.
- Personalized insights: AI will enable firms to deliver highly customized insights tailored to each client’s specific needs and challenges and so enhancing the value and impact of their services.
- Ethical AI: As AI becomes more deeply embedded in decision-making processes, firms must prioritize data ethics and transparency. This involves developing robust frameworks for responsible AI use to ensure that insights are unbiased, explainable, and compliant with regulations.
Infomineo: Transforming Data into Strategic Insights for Consultancy Firms
At Infomineo, we empower consultancy firms by leveraging AI-powered analytics to deliver actionable insights. Our expertise in both traditional data analysis and advanced AI techniques ensures that we provide tailored solutions to meet the unique needs of your organization.
Whether you’re navigating complex data landscapes or seeking to uncover hidden patterns, Infomineo’s comprehensive analytics services are designed to enhance decision-making and drive success. We integrate seamlessly with your team, using tools like Power BI and Tableau to create interactive dashboards that provide real-time insights and predictive analytics.
From optimizing supply chains to personalizing customer experiences, our solutions are built to address your specific challenges. Trust Infomineo to help you stay ahead in an increasingly data-driven world.
Frequently Asked Questions
What are the key differences between AI-powered analytics and traditional data analysis?
AI-powered analytics uses machine learning algorithms to process large, complex datasets and uncover hidden patterns. Traditional data analysis relies on statistical methods and human expertise to examine data. AI is better suited for large-scale, real-time analysis, while traditional methods are more accessible and easier to interpret.
How can consultancy firms benefit from AI-powered analytics?
AI-powered analytics enables consultancy firms to process vast amounts of data quickly and make accurate predictions. This helps firms deliver more value to clients, improve efficiency, and gain a competitive edge. AI also allows for real-time analysis and personalized insights tailored to each client’s needs.
What are the main challenges in implementing AI-powered analytics for consultancy firms?
Implementing AI-powered analytics can be complex and resource-intensive. Challenges include ensuring data quality, integrating AI with existing systems, and acquiring the necessary talent and expertise. Firms must also address data privacy and ethical concerns like bias and transparency when using AI for decision-making.
Which tools are best suited for traditional data analysis in consultancy firms?
Popular tools for traditional data analysis include Microsoft Excel, Tableau, SAS, IBM SPSS, and R. These tools offer a range of capabilities for data manipulation and statistical analysis hence making them well-suited for smaller-scale projects that don’t require advanced AI techniques.
How is AI transforming data analysis for consultancy firms?
AI is revolutionizing data analysis in the consulting industry by enabling firms to process larger datasets and make more accurate predictions. As AI becomes more prevalent, firms are moving towards a hybrid approach that combines AI with traditional methods. This shift is driving demand for new skills, technologies, and practices to support AI-powered decision-making.
Conclusion
AI-powered analytics and traditional data analysis each bring unique strengths and challenges to the table. AI-powered analytics excels at processing vast data and generating accurate predictive insights. However, it requires substantial investments, meticulous data management, and addressing interpretability and ethical concerns. On the other hand, traditional data analysis offers accessibility, interpretability, and a reliable foundation for decision-making, but may struggle with large, complex datasets and subtle patterns.
Consultancy firms must therefore carefully weigh these strengths and challenges by considering:
- Data complexity and scale
- Real-time insight requirements
- Interpretability and transparency
- Resource and expertise availability
Success might lie in a hybrid approach that blends AI’s power with traditional methods’ wisdom to deliver precise and timely insights. Firms must invest in talent, technologies, and processes while fostering collaboration and continuous learning.
The future of data analysis demands a delicate balance between AI’s potential and traditional approaches’ enduring value. Consultancy firms that strike this balance will redefine data-driven decision-making and set new benchmarks for excellence.